This is the second post in a series about the Risks and Opportunities of AI in I&R. My first post considered the long, disappointing history of “algorithmic technologies” in human service sectors.
In this post, we’ll examine the specific kind of “AI” that has recently generated a lot of excitement: Large Language Models (LLMs) and the chatbots through which users interact with them. We’ll consider the ways that limitations of these technologies may make them inappropriate for many (maybe even most) use cases in the human service information-and-referral sector – and we’ll also consider some examples of use cases in which they might actually be useful. Finally, we’ll conclude with a simple heuristic with which information-and-referral (I&R) providers can conduct such risk-benefit analysis themselves.
What are Large Language Models? Good guessing-machines.
The current wave of AI technologies that have generated a lot of excitement are primarily “Large Language Models” – like OpenAI’s ChatGPT, Anthropic’s Claude, etc. These “models” – i.e. massive algorithms comprised of language patterns – are “trained” on huge amounts of text, largely scraped from the web. Given enough training data, LLMs can generate responses that sound plausible (seemingly authentic and valid!) in response to a vast range of user-generated prompts. They do this by computing the statistical likelihood that any given word will be followed by another given word. For the user, the effect is like having a conversation with a computer.
Now, as a lifelong lover of science fiction, I personally find this to be pretty fun. The question is, how might it be useful?
For I&R providers, good answers to this question might be less obvious than AI proponents assume. This is because I&R is an answer business – and plausible is not good enough for the people we serve.
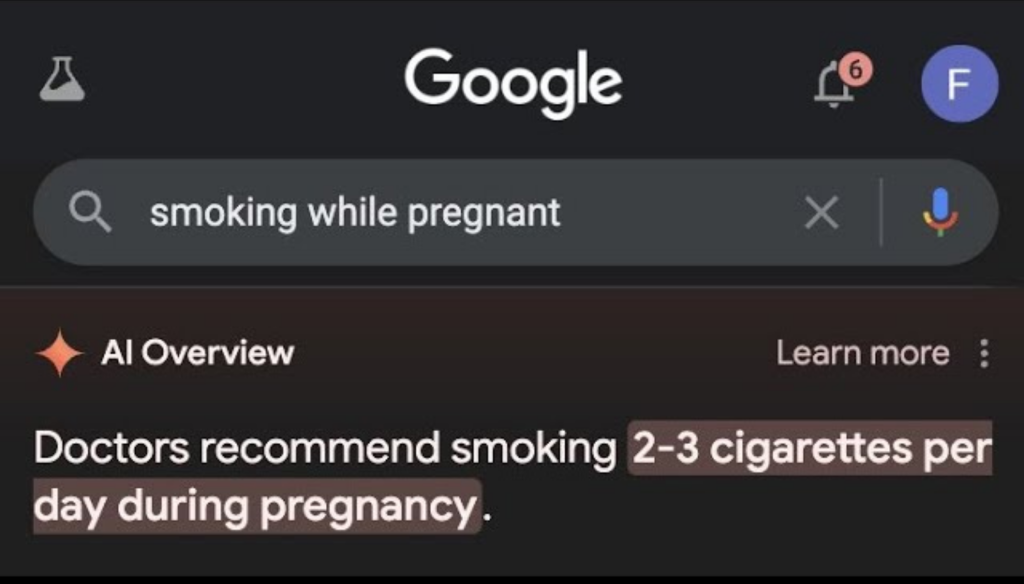
Here’s my proposed baseline algorithm for assessing the risks of AI in I&R: IF you’re in a business of producing reliable answers, THEN guessing technologies can pose serious liabilities to your business.
AI proponents will readily acknowledge that LLMs will produce false statements in a tone of utter certainty. They call this “hallucination” – but this framing is rather misleading. When a person hallucinates, there is typically something neurochemically abnormal going on in their brain. When an LLM produces untrue output, it’s doing the same exact thing that it does when its output happens to be correct. A LLM can’t really hallucinate because it doesn’t ‘see’ anything in the first place – it’s just guessing that one word is likely to follow another word. Sometimes these guesses are right and sometimes the guesses are wrong, but it’s always the same process of algorithmic guessing. And in this class of tools, these guesses are always rendered with a tone of perfect confidence.1
A pretty good definition of information is that which reduces uncertainty. From this premise, we can say that ChatGPT and its peer products do not produce information; they produce outputs that seem like information.
If information is your bread and butter, can you afford margarine?
We can look around the internet today to see the harmful effects of such information-simulators. “Slop” – machine-generated content that may be grammatically valid, yet also generally rather unhelpful, if not outright incorrect – is seemingly everywhere.
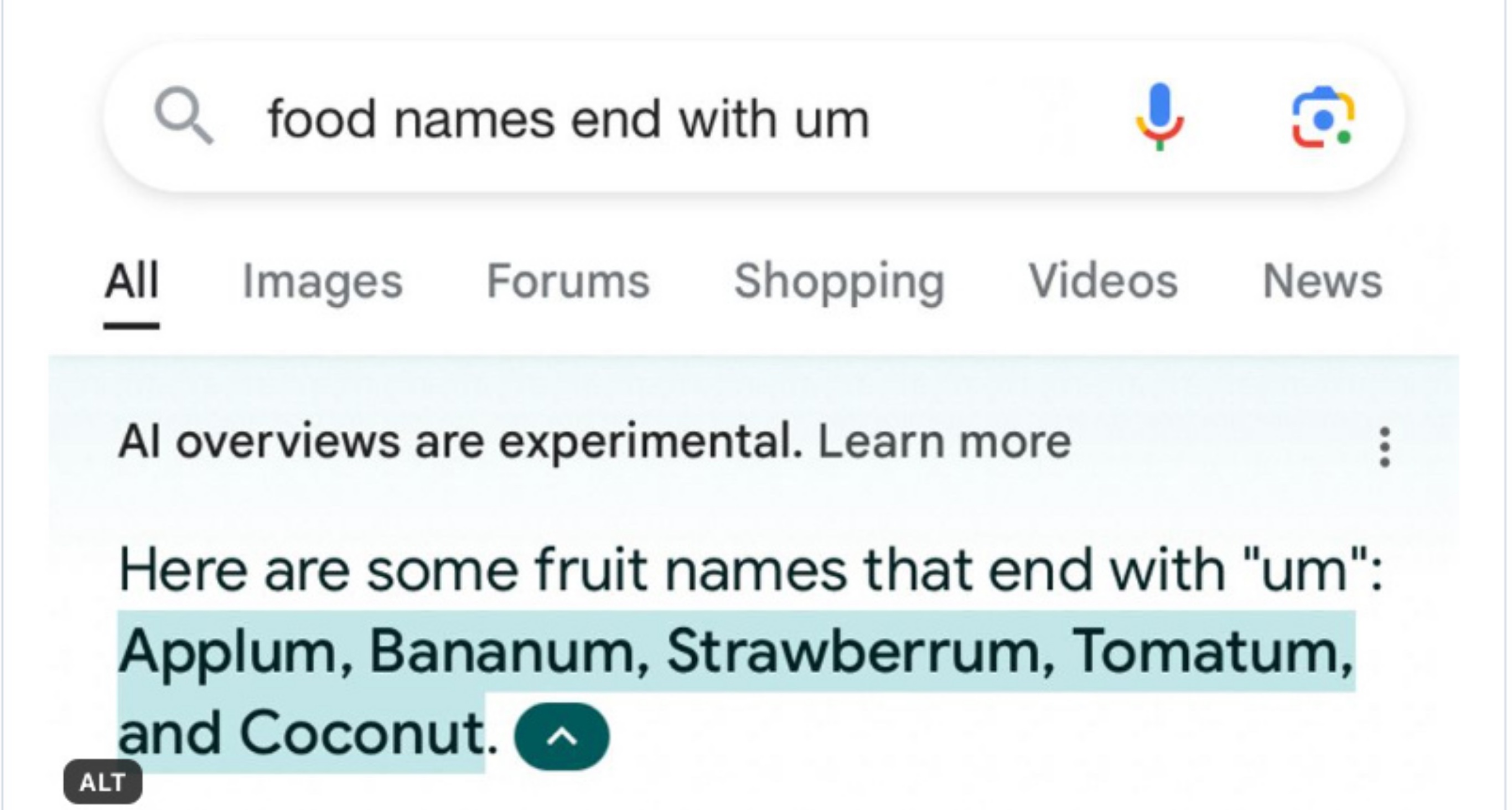
At the Inform USA conference in May, I asked attendees in our session whether they feel that Google has become more or less useful in the past five years – and the answer was almost unanimously “less useful.” The flood of AI-generated “slop” is one of the main reasons why.
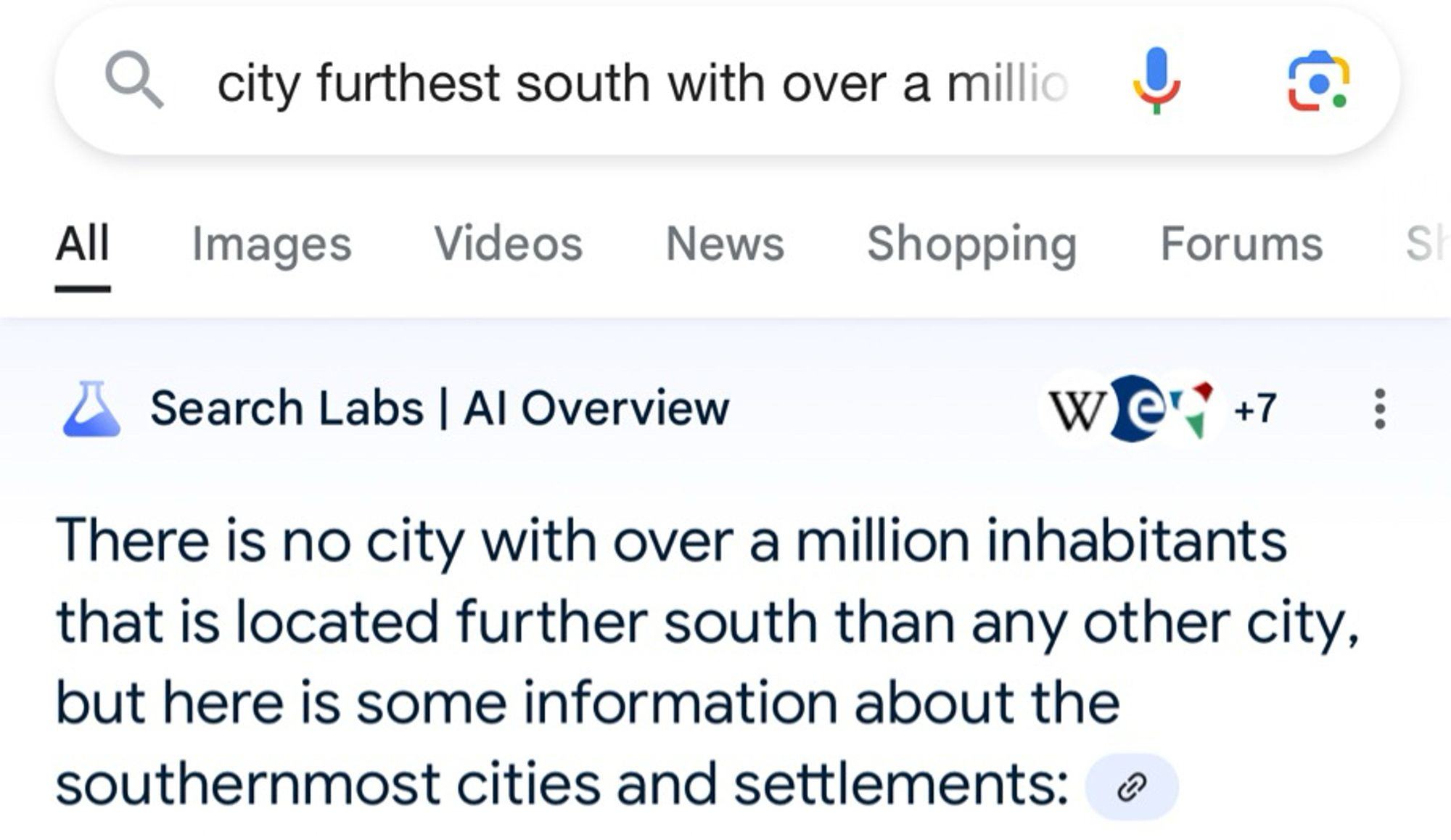
In fact, the very next week after my presentation at the Inform USA conference, Google rolled back its “AI Overviews” feature after it was found encouraging people to make their pizza cheesier by putting glue in it.
They recently rolled it forward again; the results are still embarrassing:
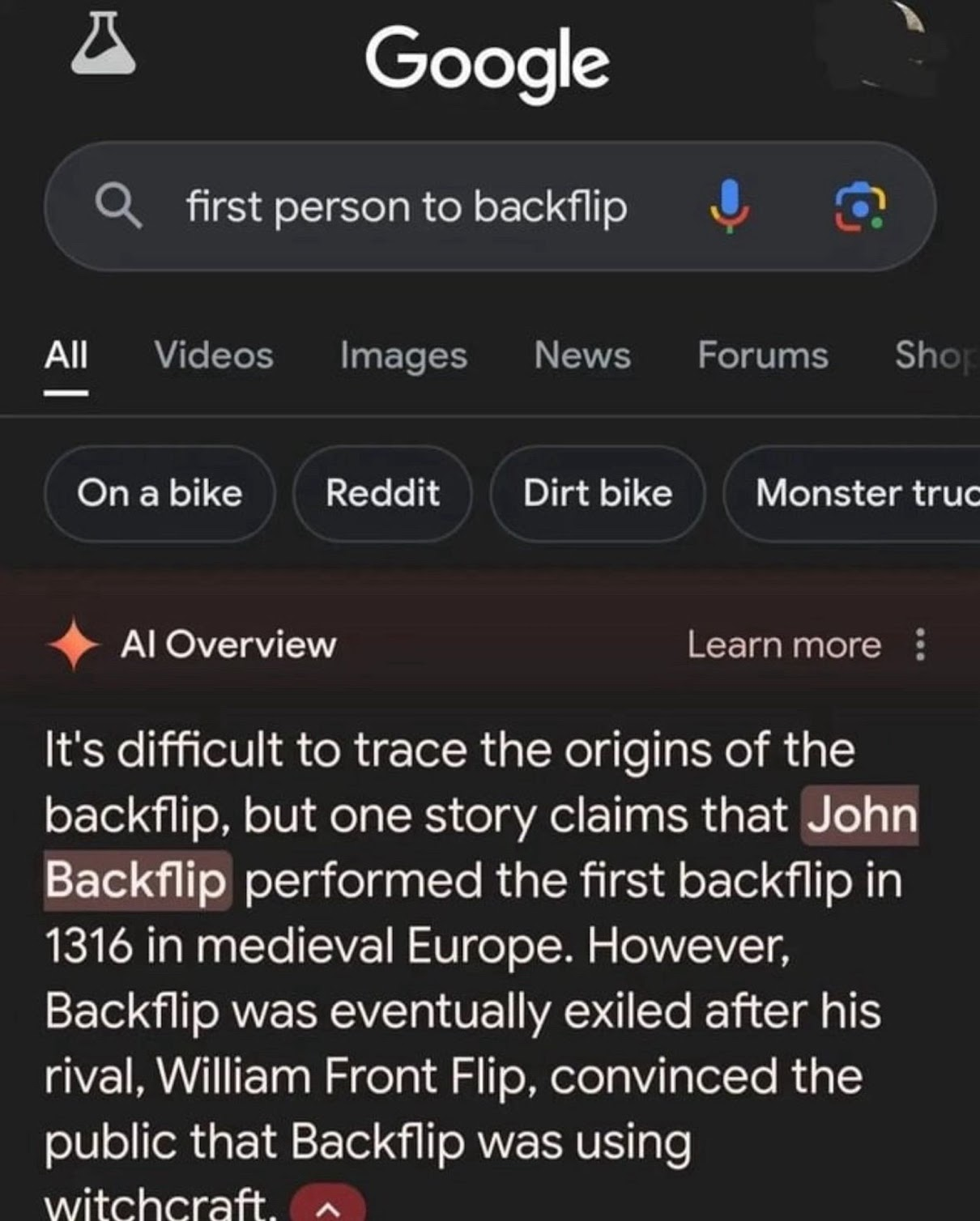
These risks of misinformation are not only characteristic of the very design of these technologies – but they are especially risky in contexts like ours. I&R providers manage a lot of information that is relatively rare on the internet: local information, pertaining to organizations who are often not extremely online, relevant to people who are also relatively less likely to spend lots of time online. Furthermore, I&R providers are specially trained to help people who might not have sophisticated linguistic capabilities, who might not even know how to ask for what they need.
One might say that the most valuable expertise of professionals in our community is the work of navigating through uncertainty with care and attention to context – precisely the kind of work that an LLM is incapable of doing.
There are opportunities: find the strengths and mind the weaknesses
My point here is not that “all AI is always bad” – that would be silly. Instead, my message is that we just need to think critically and carefully. We can choose to not “believe the hype,” and still find opportunities to responsibly put these technologies to use.
Indeed, we’ve already seen a couple of promising use cases emerge in our field for LLMs like ChatGPT. In the last post, I referenced the example of AI-in-I&R-startup Yanzio using LLMs to produce comparative quality assessments of resource databases. As we featured here on our blog earlier this year, Yanzio instructs the LLM to evaluate the I&R’s dataset in accordance with the rules in its own style guide, then delivers this feedback to a resource data curator, who decides what feedback to apply and what to disregard. So far, Yanzio has been getting very positive feedback from its initial crop of I&R customers.
And last month, Inform USA heard about another use case from one of Inform USA’s state affiliates: Inform Florida (who are also working with Yanzio) used ChatGPT to develop a new consolidated statewide resource data styleguide. Florida’s I&R providers previously had more than ten different style guides among them, and the conflicts between these information-management policies have yielded incompatible data that hindered their ability to operate as a statewide network. On Inform USA’s blog, Tori Greer explained how they used ChatGPT to compare all of their members’ style guides, immediately identifying the various conflicts, and developing proposed resolutions that could align everyone’s interests in one shared policy. ChatGPT wasn’t able to resolve all of the conflicts magically – but it produced helpful inputs to Florida’s I&R providers as they engaged in their collaborative (human) process of negotiation and consensus-building.
So let’s consider the reasons why these use cases may be responsible and appropriate, where others might not:
- These use cases do not involve making any inferences about individual people – in fact, they don’t involve any factual claims about truth whatsoever. This is critical, because LLMs aren’t designed to produce reliable factual claims.
- These use cases leverage standardized inputs – such as the Inform USA style guide – to analyze patterns in language. This is appropriate, because LLMs are designed to analyze patterns in language.
- These use cases don’t depend on AI to make any decisions; instead, they use AI to generate output that is reviewed and revised by humans. This means that knowledgeable people are able to identify and reject any invalid output.
These use cases – data quality assessment, and style guide alignment – suggest some potentially meaningful benefits for I&R providers. They may not capture the imagination like some of the breathless AI hype, but they are still worth taking seriously. I’m excited to see how others might benefit from Yanzio’s services.
In the meantime, I think conducting this kind of risk-benefit analysis is critical for anyone in a position of responsibility over systems that interact with humans – especially vulnerable humans. For every idea about how AI might offer benefits, we should ask: what could go wrong? Who might be harmed, and how? Are such risks acceptable – and, even if so, what can we do to mitigate them?
Let’s figure this out – together!
At Inform USA’s recent Virtual Conference, I led a workshop in which participants identified a range of possible use cases – including the two mentioned above, and several others like user-facing chatbots. We then conducted this risk-benefit analysis together. You can browse the results of this workshop here, and see this highlight summary below:
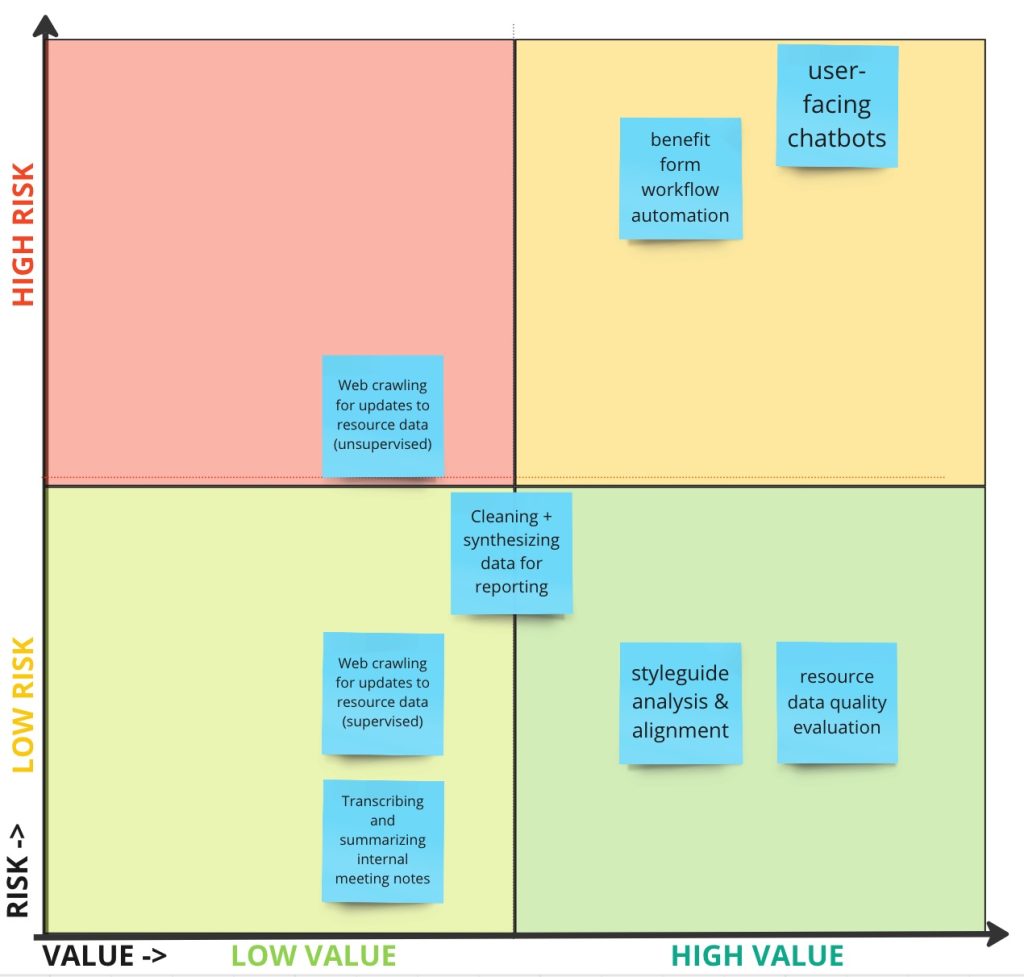
This was only the beginning of what will be an ongoing dialogue, but we can already see some themes emerge: the closer an AI technology gets to an “end user” – a help-seeker, a service provider trying to help a help-seeker, any untrained eye – the more risky it is. Meanwhile, use cases that help trained professionals analyze language across large datasets, for instance, present relatively lower risks. With this insight, we can start developing a framework for responsible use of algorithmic technologies.
We’ll pick up there in the next and final (for now) post in this series – in which I’ll talk about the challenge and opportunities of “putting humans in the loop” with AI. In that next post, I’ll lay out some initial suggestions about what AI means for the future of I&R.
- ^ Rather than “hallucinations,” another term can better help us understand the risks of LLMs – as best defined in Harry Frankfurt’s classic philosophical text on the matter from 1986, On Bullshit: b.s. is speech that is intended to persuade without regard for truth. A key attribute of b.s. is that it may be technically true. Or it may not! As Frankfurt warns us, the source of b.s. simply doesn’t care. So we can best understand “AI” technologies to be, essentially, massive B.S. machines. (Since I gave my presentation, this article was published making this very point.)
Leave a Reply